As businesses increasingly rely on artificial intelligence (AI) to drive decision-making, it's crucial that the technology is trustworthy. But what exactly does that mean? Here, we'll explore the three pillars of trustworthy AI: explainability, fairness, and security.
Pillar #1: Explainability
For AI to be trustworthy, it must be explainable. That is, users should be able to understand how the system arrived at a particular decision. If a company is using AI to decide which customers to offer credit to, for example, data scientists should be able to trace the path the system took from input (customer information) to output (decision). Otherwise, there's no way to know whether the AI is behaving as it should—or if bias has crept into its decision-making.
Pillar #2: Fairness
Fairness is closely related to explainability. To ensure that an AI system is behaving fairly, data scientists need to be able to understand how it's making decisions. If they can't track the path from input to output, they can't tell whether the system is discriminating against certain groups of people.
Pillar #3: Security
AI systems are only as secure as the data they're trained on. If that data is hacked or leaked, the resulting algorithm will be just as vulnerable. Keep in mind, too, that training data often contains sensitive information about people—information that could be used for identity theft or other malicious purposes. For this reason, it's essential that companies take steps to secure their data—and their AI systems—against potential threats.
5 Questions to Ask Before You Trust an AI Model
With all of the talk about artificial intelligence (AI), it's no wonder that business professionals are eager to implement AI solutions in their organizations. After all, AI has the potential to revolutionize nearly every industry. But before you jump on the AI bandwagon, it's important to ask some tough questions about the AI models you're considering. Here are 5 questions to ask before you trust an AI model:
- How was the AI model developed?
It's important to understand how an AI model was developed before you put your trust in it. Was the model developed by a reputable source? Was it developed using sound statistical methods? If you're not sure, be sure to consult with someone who is knowledgeable about AI development. - What data was used to train the AI model?
The data used to train an AI model is just as important as the methods used to develop it. After all, garbage in equals garbage out. Be sure to ask about the quality of the data that was used to train the model. Is it representative of the real-world data that will be fed into the model when it's deployed? - How well does the AI model generalize to new data?
Even if an AI model performs well on training data, that doesn't necessarily mean it will perform just as well on new, real-world data. That's why it's important to evaluate how well an AI model generalizes to new data before you trust it. - How transparent is the AI model?
Some organizations are reluctant to use AI because they don't understand how it works. If you're in one of those organizations, be sure to ask about the transparency of the AI models you're considering. Can you get a simple explanation of how they work? Or do they operate using a "black box" approach that makes them inscrutable? - Who is responsible for the AI model?
Finally, be sure to ask about responsibility for the AI models you're considering. Who will be responsible for monitoring them and ensuring that they operate as intended? Who will be held accountable if something goes wrong? These are important questions to consider before you put your trust in an AI solution.
Tooling to manage responsible AI
IBM recently announced IBM AI Governance is a new, one-stop solution that works with any organization's current data science platform. Included in this solution is everything needed to develop a consistent transparent model management process, capturing model development time, metadata, post-deployment model monitoring, and customized workflows.
I recommend you check AI FactSheets 360, a project from IBM research to foster trust in AI by increasing transparency and enabling governance of models. A FactSheet provides all the relevant information (facts) about the creation and deployment of AI models or services. The concept of Model Cards is also getting popular in the community, as a great way to document and provide all the key information about a Machine Learning model. For example, find here the model card of GPT-3, one of the most popular Large Language Models in the market.
The image below shows how AI Facts are captured across all the activities in the AI lifecycle for all the roles involved in the process.
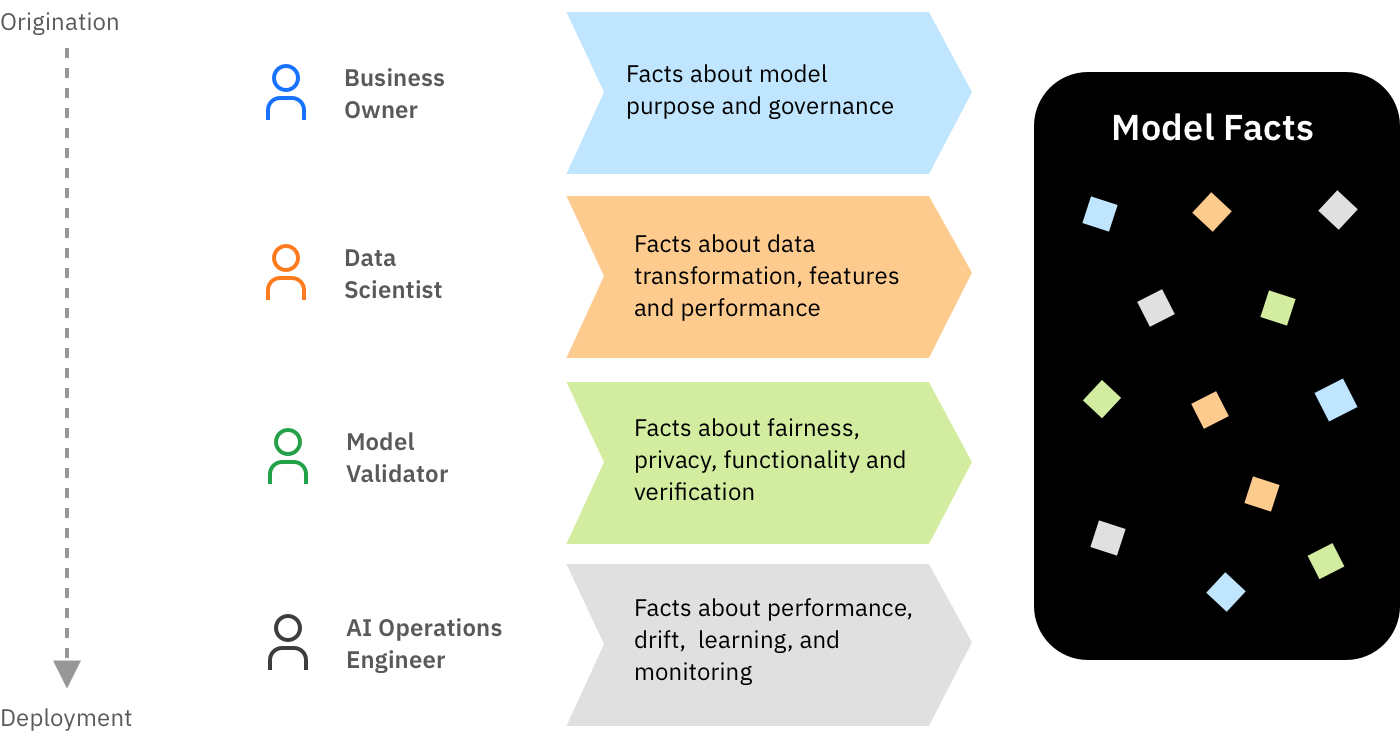
There are some great startups also in the space. For example, Credo.ai empowers organizations to create AI with the highest ethical standards. My friend Susannah Shattuck is doing a great job leading Product Management and raising the bar.
There is The Ethical AI Database project (EAIDB), the only publicly available, vetted database of AI startups providing ethical services.
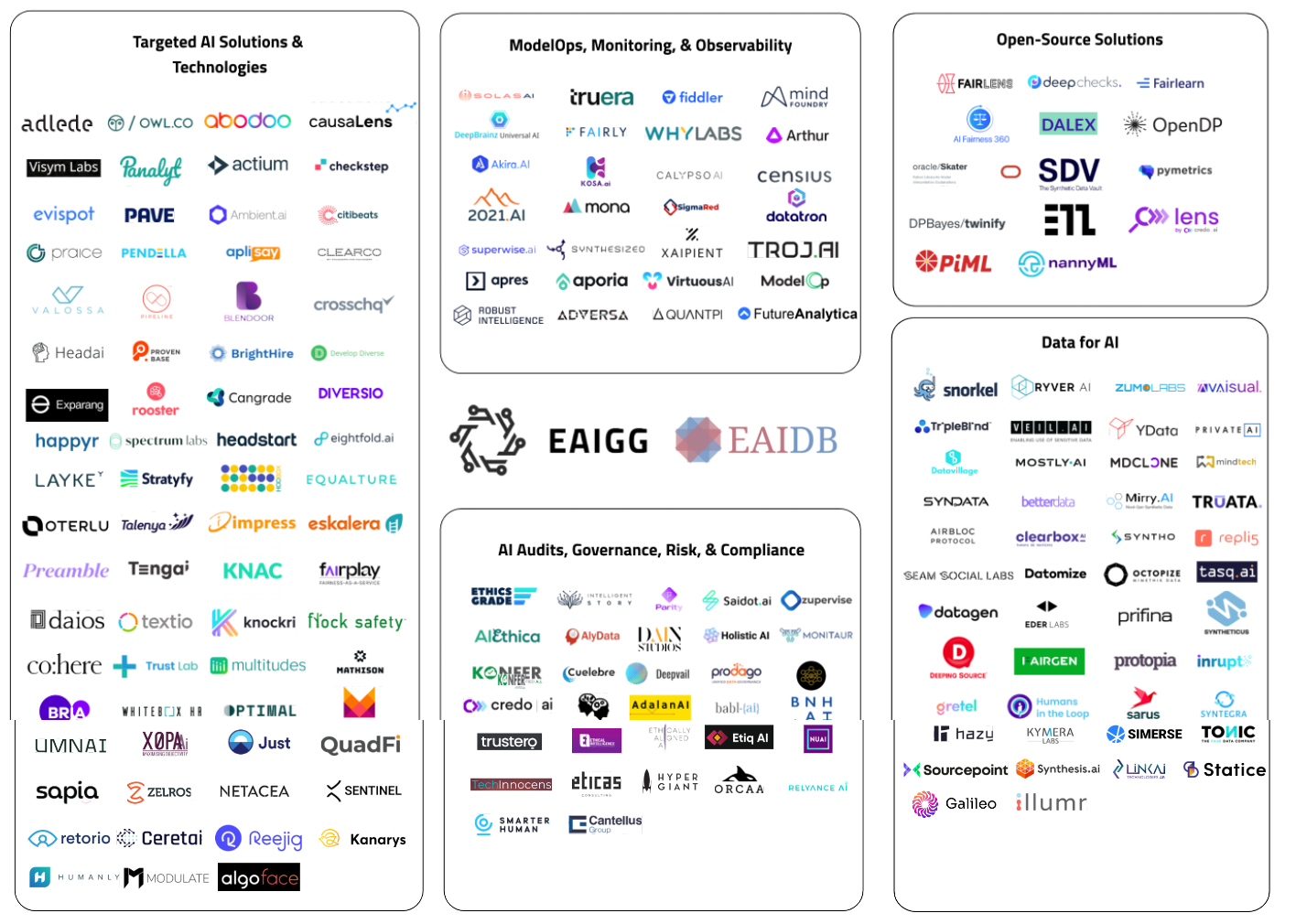
It's time to manage production AI at scale
AI has enormous potential, but it's important to ask some tough questions before implementing an AI solution in your organization. Be sure to consider how an AI model was developed, what data was used to train it, how well it generalizes the data, how transparent it is, and who is responsible for it before putting your trust in a model. Taking these factors into consideration will help ensure that you get the most out of your investment in artificial intelligence.
To build trust in AI, businesses must focus on three key areas: explainability, fairness, and security. By paying attention to these pillars of trustworthy AI, companies can help ensure that their algorithms are behaving as they should—and that they're not biased against certain groups of people. In today's data-driven world, that's more important than ever.